Data Science Training Program
Reuben Thomas
Michela Traglia
More often than not, biological experiments involve repeated measures. Examples include responses measured in cellular assays, where the repeated measures may occur over multiple experimental batches, multiple plates, or multiple wells within a plate. Observations of mouse behavior over consecutive trials would be another example of experiments involving repeated measures. The distinctions between biological and technical replicates become less clear in these scenarios. Standard statistical tests like t-tests and ANOVA are not applicable. Instead, analysis of such data requires more sophisticated approaches, such as Linear Mixed Effect Modeling.
In this course, you will learn the motivation for the use of these models, the underlying description and assumptions behind them, example scenarios where they can be used, and code to implement and interpret these models in R.
Visit the workshop site for more details and materials.
Details
Dates
1:00pm–3:00pm
1:00pm–3:00pm
Location
OnlineContact(s)
The Gladstone Data Science Training Program was started in 2018 to provide trainees with learning opportunities and hands-on workshops to improve their skills in bioinformatics and computational analysis. This program offers a series of workshops throughout the year to enable trainees to gain new skills and get support with their questions and data.
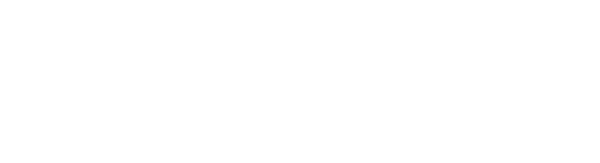
Diversity, Equity, and Inclusion
At Gladstone, we are committed to providing events and professional development activities that resonate with our community’s diverse members. Our goal is to develop creative programming that encompasses a wide variety of ideas and perspectives to inspire, educate, and engage with everyone within our walls.
We want to effect positive change through our events and activities by providing a platform for discussions on important topics related to increasing diversity and inclusiveness in the sciences.