Barbara Engelhardt works to improve human health by analyzing the enormous reams of data generated by research labs, doctors, and hospitals. Combining mathematical, statistical, and artificial intelligence approaches, her team seeks to understand the cellular mechanisms of disease, the impact of traumatic life events on health, and the clinical treatments that best correlate with positive health outcomes. The team anchors their work in a large network of collaborations, and generates computational and statistical tools aimed at increasing the impact of both medical research and healthcare practice.
Disease Areas
Areas of Expertise
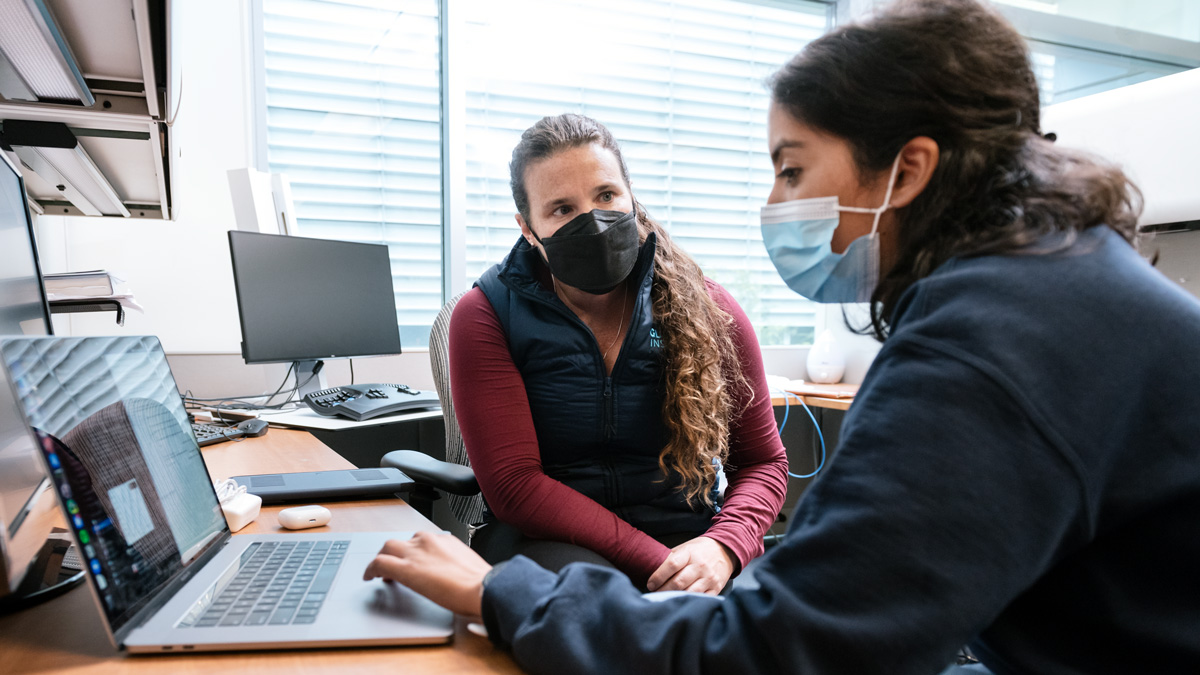
Lab Focus
Research Impact
Medical research has seen an explosion of genomic, single-cell, and imaging data that can only be mined productively with structured and robust statistical and computational approaches. Similarly, modern clinical practice is generating vast troves of electronic records on patients, interventions, and health outcomes that could be used to predict the best course of treatment or to correct health inequities.
Barbara Engelhardt has been tackling these challenges with a combination of statistics and machine learning approaches. Her analysis of electronic health care data has led to a policy for weaning patients from a mechanical ventilator, and another that reduced the number of blood draws for hospital tests by 40 percent and accelerated the diagnosis of sepsis by approximately four hours.
Her work in genomics seeks to understand the genetic basis for complex traits and the cellular mechanisms underlying this relationship. Complex traits, including many diseases, manifest as a continuum of symptoms that reflect dysregulation among cell types, genes, and other factors. Engelhardt has developed tools to explore, identify, and quantify these interactions in large genomic datasets, and to predict the impact of specific interventions. In particular, she is pursuing approaches to incorporate the quantitative output of genes (how much RNA they produce) into her analysis of complex traits, which has led her to identify a gene that protects from muscular myopathy in the context of statin treatment. She is also developing tools to extract biological or disease-relevant information from time series gene expression data, and to identify optimal gene markers to improve the analysis of omic data from single cells.
Professional Titles
Senior Investigator, Gladstone Institutes
Professor, Department of Biomedical Data Science, Stanford University
Bio
Barbara Engelhardt, PhD, is a senior investigator at Gladstone Institutes. She is also a professor in the Department of Biomedical Data Science at Stanford University.
Engelhardt opened her lab at Gladstone in 2021. Prior to joining Princeton in 2014, she was an assistant professor in biostatistics and bioinformatics and statistical sciences at Duke University. She graduated from Stanford University, received her PhD in electrical engineering and computer science from UC Berkeley, supported by an NSF Graduate Research Fellowship, and trained as a postdoctoral researcher at the University of Chicago. Engelhardt also spent 2 years working at Jet Propulsion Laboratory, a summer at Google Research, and a year at 23andMe.
Her research interests involve developing statistical models and methods for the analysis of high-dimensional biomedical data, with a goal of understanding the underlying biological mechanisms of complex phenotypes and human disease.
Engelhardt received the 2021 Overton Prize from the International Society for Computational Biology, one of the top awards in this field.
Honors and Awards
2021 Overton Prize, International Society for Computational Biology
2020–2021 Fast Grants for Covid-19 Research
2019–2020 Schmidt DataX Project Award (with Toettcher Lab), Princeton University
2018–2022 Faculty Early Career Development (CAREER) Award, National Science Foundation
2018–2019 Grants for the Human Cell Atlas, Silicon Valley Community Foundation and Chan Zuckerberg Initiative
2017–2019 Helen Shipley Hunt Fund Award, Princeton University
2016–2018 Sloan Research Fellowship, Alfred P. Sloan Foundation
2016 E. Lawrence Keyes, Jr./Emerson Electric Co. Faculty Advancement Award, Princeton University
2015 J. Blair Pyne Fund Award, Princeton University
2013 DIBS Research Incubator Awards, Duke Institute for Brain Sciences
2011–2015 K99 Pathway to Independence Career Award, National Human Genome Research Institute
2005–2006 Google Anita Borg Memorial Scholarship
2004 Walter M. Fitch Prize, Society for Molecular Biology and Evolution
2001 Graduate Research Fellowship, National Science Foundation
Publications
- Hierarchical Gaussian processes and mixtures of experts to model Covid-19 patient trajectories. Cui S, Yoo EC, Li D, Laudanski K, Engelhardt BE. Proceedings of the Pacific Symposium on Biocomputing. 2022 (accepted).
- Brain kernel: a new spatial covariance function for fMRI data. Wu A, Nastase SA, Baldassano CA, Turk-Browne NB, Norman KA, Engelhardt BE, Pillow JW. NeuroImage. 2021 (accepted).
- Causal network inference from gene transcriptional time series response to glucocorticoids. Lu J, Dumitrascu B, McDowell IC, Jo B, Barrera A, Hong L, Leichter SM, Reddy TE, Engelhardt BE. PLoS Comput Biol. 2021 Jan 29;17(1):e1008223.
- Joint analysis of gene expression levels and histological images identifies genes associated with tissue morphology. Ash J, Darnell G, Munro D, Engelhardt BE. Nat Commun. 2021 Mar 11;12(1):1609.
- Optimal marker gene selection for cell type discrimination in single cell analyses. Dumitrascu B, Villar S, Mixon DG, Engelhardt BE. Nat Commun. 2021 Feb 19;12(1):1186.
- Active multi-fidelity Bayesian online changepoint detection. Gundersen GW, Cai D, Zhou C, Engelhardt BE, Adams, RP. Uncertainty in Artifical Intelligence. 2021.
- A self-exciting point process to study multi-cellular spatial signaling patterns. Verma A, Jena SG, Isakov DR, Aoki K, Toettcher JE, Engelhardt BE Proc Natl Acad Sci USA. 2021 Aug 10;118(32):e2026123118.
- Contrastive latent variable modeling with application to case-control sequencing experiments. Jones A, Townes WF, Li D, Engelhardt BE. Annals of Applied Statistics. 2021 (accepted).
- COP-E-CAT: Cleaning and organization pipeline for EHR computational and analytic tasks. Mandyam A, Yoo EC, Soules J, Laudanski K, Engelhardt BE. Proceedings of the 12th ACM Conference on Bioinformatics, Computational Biology, and Health Informatics. 2021.
- Latent variable modeling with random features. Gundersen GW, Zhang MM, Engelhardt BE. Artificial Intelligence and Statistics. 2021.
- Defining admissible rewards for high confidence policy evaluation. Prasad N, Engelhardt BE, Doshi-Velez F. ACM Conference on Health, Inference, and Learning. 2020.
- Patient-specific effects of medication using latent force models with Gaussian processes. Cheng L, Dumitrascu B, Zhang MM, Chivers C, Draugelis ME, Li K, Engelhardt BE. Artificial Intelligence and Statistics. 2020.
- Measuring the predictability of life outcomes with a scientific mass collaboration. Salganik M, et al. Proc Natl Acad Sci USA. 2020 Apr 14;117(15):8398-8403.
- Sparse multi-output Gaussian processes for medical time series prediction. Cheng L, Dumitrascu B, Darnell G, Chivers C, Draugelis M, Li K, Engelhardt BE. BMC Med Inform Decis Mak. 2020 Jul 8;20(1):152.
- Nonparametric Bayesian multi-armed bandits for single cell experiment design. Camerlenghi F, Dumitrascu B, Ferrari F, Engelhardt BE, Favaro S. Annals of Applied Statistics. 2020.
- The impact of sex on gene expression across human tissues. Oliva M, GTEx Consortium, et al. Science. 2020 Sep 11;369(6509):eaba3066.
- The GTEx Consortium atlas of genetic regulatory effects across human tissues. GTEx Consortium. Science. 2020 Sep 11;369(6509):1318-1330.
- ACE inhibition and cardiometabolic risk factors, lung ACE2 and TMPRSS2 gene expression, and plasma ACE2 levels: a Mendelian randomization study. Gill, D, Arvanitis M, Carter P, Cordero AHI, Jo B, Karhunen V, Larsson SC, Li X, Lockhart SM, Mason A, Pashos E, Saha A, Tan VY, Zuber V, Bossé Y, Fahle S, Hao K, Jiang T, Joubert P, Lunt AC, Ouwehand WH, Roberts DJ, Timens W, van den Berge M, Watkins NA, Battle A, Butterworth AS, Danesh J, Angelantonio ED, Engelhardt BE, Peters JE, Sin DD, Burgess S. R Soc Open Sci. 2020 Nov 18;7(11):200958.
- A robust nonlinear low-dimensional manifold for single cell RNA-seq data. Verma A, Engelhardt BE. BMC Bioinformatics. 2020 Jul 21;21(1):324.
- netNMF-sc: Leveraging gene-gene interactions for imputation and dimensionality reduction in single-cell expression analysis. Elyanow R, Dumitrascu B, Engelhardt BE, Raphael BJ. Genome Res. 2020 Feb;30(2):195-204.
- An optimal policy for patient laboratory tests in intensive care units. Cheng L, Prasad N, Engelhardt BE. Pac Symp Biocomput. 2019;24:320-331.
- Predicting sick patient volume in a pediatric outpatient setting using time series analysis. Guan G, Engelhardt BE. Proceedings of Machine Learning for Health Care. 2019.
- netNMF-sc: Leveraging gene-gene interactions for imputation and dimensionality reduction in single-cell expression analysis. Elyanow R, Dumitrascu B, Engelhardt BE, Raphael BJ. Proceedings of the 23rd International Conference on Research in Computational Molecular Biology. 2019.
- End-to-end training of deep probabilistic CCA on paired biomedical observations. Gundersen G, Dumitrascu B, Engelhardt BE. Proceedings of the Conference on Uncertainty in Artificial Intelligence. 2019
- Clustering gene expression time series data using an infinite Gaussian process mixture model. McDowell IC, Manandhar D, Vockley CM, Schmid AK, Reddy TE, Engelhardt BE. PLoS Comput Biol. 2018 Jan 16;14(1):e1005896.
- Statistical tests for detecting variance effects in quantitative trait studies. Dumitrascu B, Darnell G, Ayroles J, Engelhardt BE. Bioinformatics. 2019 Jan 15;35(2):200-210.
- Glucocorticoid receptor recruits to enhancers and drives activation by motif-directed binding. McDowell IC, Barrero A, D’Ippolito AM, Vockley CM, Hong LK, Leichter SM, Bartelt LC, Majoros WH, Song L, Safi A, Koçak DD, Gersbach CA, Hartemink AJ, Crawford GE, Engelhardt BE, Reddy TE. Genome Res. 2018 Sep;28(9):1272-1284.
- BIISQ: Bayesian nonparametric discovery of Isoforms and Individual Specific Quantification. Aguiar D, Cheng L, Dumitrascu B, Mordelet F, Pai AA, Engelhardt BE. Nat Commun. 2018 Apr 27;9(1):1681.
- How algorithmic confounding in recommendation systems increases homogeneity and decreases utility. Chaney AJB, Stewart BM, Engelhardt BE. 12th ACM Conference on Recommender Systems. 2018.
- PG-TS: Improved Thompson sampling for logistic contextual bandits. Dumitrascu B, Feng K, Engelhardt BE. Proceedings of Neural Information Processing Systems. 2018.
- Computational approaches to fMRI analysis. Cohen JD, Daw N, Engelhardt B, Hasson U, Li K, Niv Y, Norman KA, Pillow J, Ramadge PJ, Turk-Browne NB, Willke TL. Nat Neurosci. 2017 Feb 23;20(3):304-313.
- Expandable factor analysis. Srivastava S, Engelhardt BE, Dunson DB. Biometrika. 2017 Sep;104(3):649-663.
- Genetic effects on gene expression across human tissues. GTEx Consortium, Battle A, Brown CD, Engelhardt BE, Montgomery SM. Nature. 2017 Oct 11;550(7675):204-213.
- Fast moment estimation for generalized latent Dirichlet models. Zhao S, Engelhardt BE, Mukherjee S, Dunson DB. Journal of the American Statistical Association. 2017.
- Adaptive randomized dimension reduction on massive data. Darnell G, Georgiev S, Mukherjee S, Engelhardt BE. Journal of Machine Learning Research. 2017;18(140):1-30.
- Co-expression networks reveal the tissue-specific regulation of transcription and splicing. Saha A, Kim Y, Gewirtz ADH, Jo B, Gao C, McDowell IC, GTEx Consortium, Engelhardt BE, Battle A. Genome Res. 2017 Nov;27(11):1843-1858.
- A reinforcement learning approach to weaning of mechanical ventilation in intensive care units. Prasad N, Cheng L, Chivers C, Draugelis M, Engelhardt BE. Proceedings of Uncertainty in Artificial Intelligence. 2017;1-9.
- Dynamic collaborative filtering with compound Poisson factorization. Jerfel G, Basbug ME, Engelhardt BE. Proceedings of Artificial Intelligence and Statistics. 2017;54:738-747.
- Hierarchical compound Poisson factorization. Basbug ME, Engelhardt BE. Proceedings of the International Conference on Machine Learning. 2016; 1795-1803.
- Context specific and differential gene co-expression networks via Bayesian biclustering. Gao C, McDowell IC, Zhao S, Brown CD, Engelhardt BE. PLoS Comput Biol. 2016 Jul 28;12(7):e1004791.
- Detecting differential growth of microbial populations with Gaussian process regression. Tonner PD, Darnell CL, Engelhardt BE, Schmid AK. Genome Res. 2017 Feb;27(2):320-333.
- Bayesian group latent factor analysis with structured sparse priors. Zhao S, Gao C, Mukherjee S, Engelhardt BE. Journal of Machine Learning Research. 2016.
Contact
Barbara Engelhardt
Email
415.734.2503
Liz Nguyen
Administrative Associate
415.734.2675
Email